Coronavirus outbreak
'There is no absolute truth': an infectious disease expert on Covid-19, misinformation and 'bullshit'
Carl Bergstrom’s two disparate areas of expertise merged as reports of a mysterious respiratory illness emerged in January
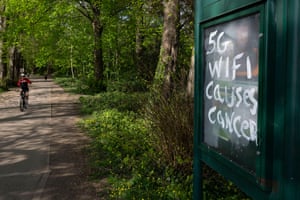
Julia Carrie Wong in Oakland
Tue 28 Apr 2020 11.00 BST
C
arl Bergstrom is uniquely suited to understanding the current moment. A professor of biology at the University of Washington, he has spent his career studying two seemingly disparate topics: emerging infectious diseases and networked misinformation. They merged into one the moment reports of a mysterious respiratory illness emerged from China in January.
The coronavirus touched off both a pandemic and an “infodemic” of hoaxes, conspiracy theories, honest misunderstandings and politicized scientific debates. Bergstrom has jumped into the fray, helping the public and the press navigate the world of epidemiological models, statistical uncertainty and the topic of his forthcoming book: bullshit.
The following interview has been edited for length and clarity.
You’ve been teaching a course and have co-written a book about the concept of bullshit. Explain what you mean by bullshit?
The formal definition that we use is “language, statistical figures, data, graphics and other forms of presentation that are intended to persuade by impressing and overwhelming a reader or listener with a blatant disregard for truth or logical coherence”.
The idea with bullshit is that it’s trying to appear authoritative and definitive in a way that’s not about communicating accurately and informing a reader, but rather by overwhelming them, persuading them, impressing them. If that’s done without any allegiance to truth, or accuracy, that becomes bullshit.
We’re all used to verbal bullshit. We’re all used to campaign promises and weasel words, and we’re pretty good at seeing through that because we’ve had a lot of practice. But as the world has become increasingly quantified and the currency of arguments has become statistics, facts and figures and models and such, we’re increasingly confronted, even in the popular press, with numerical and statistical arguments. And this area’s really ripe for bullshit, because people don’t feel qualified to question information that’s given to them in quantitative form.
Are there bullshit narratives about the coronavirus that you are concerned about right now?
What’s happened with this pandemic that we’re not accustomed to in the epidemiology community is that it’s been really heavily politicized. Even when scientists are very well-intentioned and not trying to support any side of the narrative, when they do work and release a paper it gets picked up by actors with political agendas.
Whether it’s talking about seroprevalence or estimating the chance that this is even going to come to the United States at all each study gets picked up and placed into this little political box and sort of used as a cudgel to beat the other side with.
So even when the material isn’t being produced as bullshit, it’s being picked up and used in the service of that by overstating its claims, by cherry-picking the information that’s out there and so on. And I think that’s kind of the biggest problem that we’re facing.
One example [of intentional bullshit] might be this insistence for a while on graphing the number of cases on a per-capita basis, so that people could say the US response is so much better than the rest of the world because we have a slower rate of growth per capita. That was basically graphical malfeasance or bullshit. When a wildfire starts spreading, you’re interested in how it’s spreading now, not whether it’s spreading in a 100-acre wood or millions of square miles of national forest.
Is there one big lesson that you think that the media should keep in mind as we communicate science to the public? What mistakes are we making?
I think the media has been adjusting really fast and doing really well. When I’m talking about how to avoid misinformation around this I’m constantly telling people to trust the professional fact-based media. Rather than looking for the latest rumor that’s spreading across Facebook or Twitter so that you can have information up to the hour, recognize that it’s much better to have solidly sourced, well-vetted information from yesterday.
Hyper-partisan media are making a huge mess of this, but that’s on purpose. They’ve got a reason to promote hydroxychloroquine or whatever it is and just run with that. They’re not even trying to be responsible.
But one of the biggest things that people [in the media] could do to improve would be to recognize that scientific studies, especially in a fast-moving situation like this, are provisional. That’s the nature of science. Anything can be corrected. There’s no absolute truth there. Each model, each finding is just adding to a weight of evidence in one direction or another.
A lot of the reporting is focusing on models, and most of us probably don’t have any basic training in how to read them or what kind of credence to put in them. What should we know?
The key thing, and this goes for scientists as well as non-scientists, is that people are not doing a very good job thinking about what the purpose of different models are, how the purposes of different models vary, and then what the scope of their value is. When these models get treated as if they’re oracles, then people both over-rely on them and treat them too seriously – and then turn around and slam them too hard for not being perfect at everything.
Are there mistakes that are made by people in the scientific community when it comes to communicating with the public?
We’re trying to communicate as a scientific community in a new way, where people are posting their data in real time. But we weren’t ready for the degree to which that stuff would be picked up and assigned meaning in this highly politically polarized environment. Work that might be fairly easy for researchers to contextualize in the field can be portrayed as something very, very different in the popular press.
The first Imperial College model in March was predicting 1.1 million to 2.2 million American deaths if the pandemic were not controlled. That’s a really scary, dramatic story, and I still think that it’s not unrealistic. That got promoted by one side of the partisan divide. Then Imperial came back and modeled a completely different scenario, where the disease was actually brought under control and suppressed in the US, and they released a subsequent model that said, ‘If we do this, something like 50,000 deaths will occur.’ That was picked up by the other side and used to try to discredit the Imperial College team entirely by saying, ‘A couple of weeks ago they said a million now they’re saying 50,000; they can’t get anything right.’ And the answer , of course, is that they were modeling two different scenarios.
We’re also not doing enough of deliberately stressing the possible weaknesses of our interpretations. That varies enormously from researcher to researcher and team to team.
It requires a lot of discipline to argue really hard for something but also be scrupulously open about all of the weaknesses in your own argument.
But it’s more important than ever, right? A really good paper will lay out all the most persuasive evidence it can and then in the conclusion section or the discussion section say, ‘OK, here are all the reasons that this could be wrong and here are the weaknesses.’
When you have something that’s so directly policy relevant, and there’s a lot of lives at stake, we’re learning how to find the right balance.
It is a bit of a nightmare to put out data that is truthful, but also be aware that there are bad faith actors at the moment who might pounce on it and use it in a way you didn’t intend.
There’s a spectrum. You have outright bad faith actors – Russian propaganda picking up on things and bots spreading misinformation – and then you have someone like Georgia Governor Brian Kemp who I wouldn’t call a bad faith actor. He’s a misinformed actor.
There’s so much that goes unsaid in science in terms of context and what findings mean that we don’t usually write in papers. If someone does a mathematical forecasting model, you’re usually not going to have a half-page discussion on the limitations of forecasting. We’re used to writing for an audience of 50 people in the world, if we’re lucky, who have backgrounds that are very similar to our own and have a huge set of shared assumptions and shared knowledge. And it works really well when you’re writing on something that only 50 people in the world care about and all of them have comparable training. But it is a real mess when it becomes pressing, and I don’t think any of us have figured out exactly what to do about that because we’re also trying to work quickly and it’s important to get this information out.
One area that has already become contentious and in some ways politicized is the serology surveys, which are supposed to show what percentage of the population has antibodies to the virus. What are some of the big picture contextual caveats and limitations that we should keep in mind as these surveys come out?
The seroprevalence in the US is a political issue, and so the first thing is to recognize that when anyone is reporting on that stuff, there’s a political context to it. It may even be that some of the research is being done with an implicitly political context, depending on who the funders are or what the orientations and biases of some of the researchers.
On the scientific side, I think there’s really two things to think about. The first one is the issue of selection bias. You’re trying to draw a conclusion about one population by sampling from a subset of that population and you want to know how close to random your subset is with respect to the thing you’re trying to measure. The Santa Clara study recruited volunteers off of Facebook. The obvious source of sampling bias there is that people desperately want to get tested. The people that want it are, of course, people that think they’ve had it.
The other big piece is understanding the notion of positive predictive value and the way false positive and false negative error rates influence the estimate. And that depends on the incidence of infection in the population.
If you have a test that has a 3% error rate, and the incidence in the population is below 3%, then most of the positives that you get are going to be false positives. And so you’re not going to get a very tight estimate about how many people have it. This has been a real problem with the Santa Clara study. From my read of the paper, their data is actually consistent with nobody being infected. A New York City study on the other hand showed 21% seropositive, so even if there has a 3% error rate, the majority of those positives have to be true positives.
Now that we’ve all had a crash course in models and serosurveys, what are the other areas of science where it makes sense for the public to start getting educated on the terms of the debate?
One that I think will come along sooner or later is interpreting studies of treatments. We’ve dealt with that a little bit with the hydroxychloroquine business but not in any serious way because the hydroxychloroquine work has been pretty weak and the results have not been so positive.
But there are ongoing tests of a large range of existing drugs. And these studies are actually pretty hard to do. There’s a lot of subtle technical issues: what are you doing for controls? Is there a control arm at all? If not, how do you interpret the data? If there is a control arm, how is it structured? How do you control for the characteristics of the population on whom you’re using the drug or their selection biases in terms of who’s getting the drug?
Unfortunately, given what we’ve already seen with hydroxychloroquine, it’s fairly likely that this will be politicized as well. There’ll be a parallel set of issues that are going to come around with vaccination, but that’s more like a year off.
If you had the ability to arm every person with one tool – a statistical tool or scientific concept – to help them understand and contextualize scientific information as we look to the future of this pandemic, what would it be?
I would like people to understand that there are interactions between the models we make, the science we do and the way that we behave. The models that we make influence the decisions that we take individually and as a society, which then feed back into the models and the models often don’t treat that part explicitly.
Once you put a model out there that then creates changes in behavior that pull you out of the domain that the model was trying to model in the first place. We have to be very attuned to that as we try to use the models for guiding policy.
That’s very interesting, and not what I expected you to say.
What did you expect?
That correlation does not imply causation.
That’s another very good one. Seasonality is a great example there. We’re trying a whole bunch of things at the same time. We’re throwing all kinds of possible solutions at this and lots of things are changing. It’s remarkable to me actually, that so many US states are seeing the epidemic curve decrease. And so there’s a bunch of possibilities there. It could be because people’s behavior is changing. There could be some seasonality there. And there are other possible explanations as well.
But what is really important is that just because the trend that you see is consistent with a story that someone’s selling, there may be many other stories that are also consistent, so inferring causality is dangerous.
- Calling Bullshit: The Art of Scepticism in a Data-Driven World by Carl Bergstrom and Jevin West will be published 4 August 2020 by Penguin.
Carl Bergstrom
Geen opmerkingen:
Een reactie posten
Opmerking: Alleen leden van deze blog kunnen een reactie posten.